There is a constant stream of emerging innovations in Agtech, and one of the biggest topics is Machine Learning and Artificial Intelligence (AI). This is currently a hot topic out in the field and behind a computer.
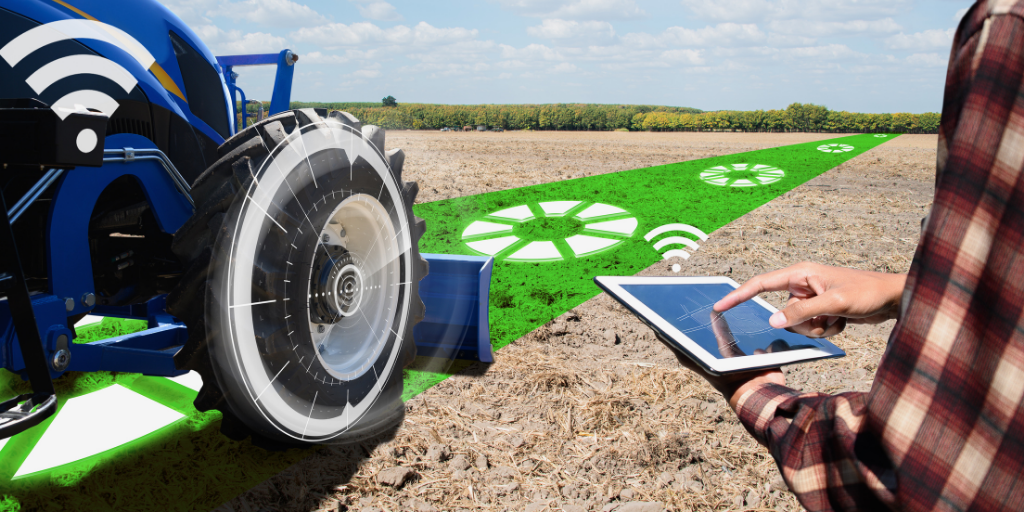
This buzz is happening with good reason. AI and machine learning are changing every industry for the better these days.
According to MIT Computer Science Professor Aleksander Madry, "Machine learning is changing, or will change, every industry, and leaders need to understand the basic principles, the potential, and the limitations."
The good news is that these autonomous fruit picking machines are getting more accurate, more efficient, and smarter every week. A few apple orchard robot pickers are even ready for action right now and within the next five years, we could see worldwide large-scale adoption of some of this technology. There are many automated fruit harvesting machines that are being developed and potentially many options for a grower to consider trialing or purchasing.
But there is some confusion about what these two complicated tech concepts are, especially when it comes to agrotech applications.
Croptracker will show you what it is happening on the tech side of agriculture and how AIs are deployed today.
Machine Learning and Artificial Intelligence in Agriculture: What's the difference?
When companies use AI to replace existing operations or provide customers with these helpful and powerful tools, they are most likely using machine learning.
According to IBM, "Machine learning is a branch of artificial intelligence (AI) and computer science which focuses on the use of data and algorithms to imitate the way that humans learn, gradually improving its accuracy." This is a complicated way of saying the machine does literally learn from the information it is given and becomes a replacement or substitute for skilled knowledge from humans.
Artificial Intelligence, on the other hand, "refers to systems or machines that mimic human intelligence to perform tasks and can iteratively improve themselves based on the information they collect."
This means not all forms of artificial intelligence are machine learning, but all forms of machine learning are AI.
In other words, artificial intelligence has many parts to it, and machine learning is just one of them. Most of the applications we see in our daily lives, including Netflix movie suggestions and Google's search engine, use machine learning to show you accurate information.
How Does Machine Learning Help Growers?
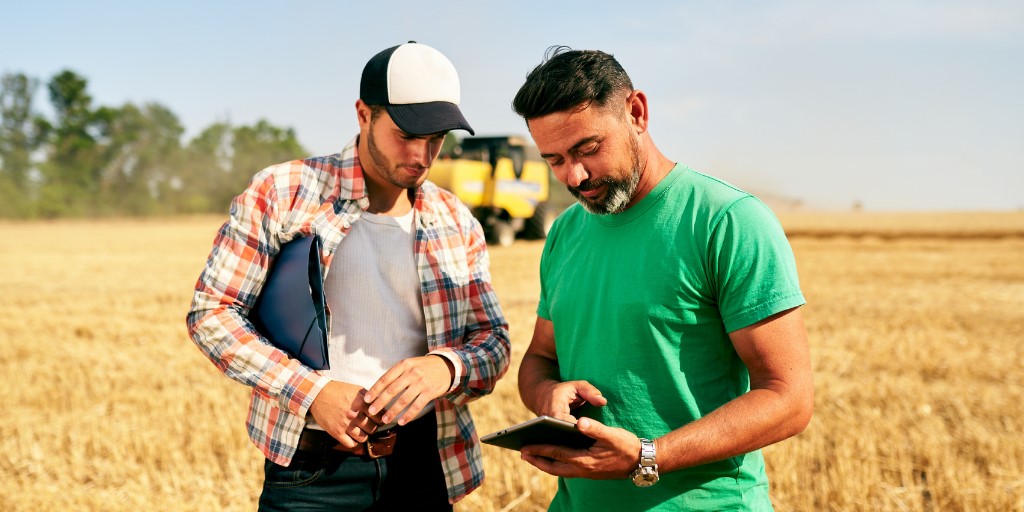
Machine learning has massive potential to disrupt every part of the agriculture industry in the next 100 years. It is showing up in everything from drones learning how to navigate apple orchards to farm management software.
At the end of the day, these technologies increase profits by automating repetitive tasks and using the computer to compute massive data sets.
AIs help farmers focus on the important things at their farm. They do not have to try to figure out details and remember loads of information at a later date. An AI tool does not guess or react to intuition based on years of experience like an experienced farmer does. But it can incorporate knowledge from the farm to do a task that growers need done.
There is another significant advantage over traditional methods. Nothing is forgotten by the computer's memory unless you tell it to. There is no need to search for lost papers. Managers and bosses do not have to ask a worker who knows the information to try and remember an important detail that wasn’t important months earlier.
Machine learning can help farmers make informed management decisions on what to grow towards matching the crop to the existing market's demands.
Smart farming is a concept that has been developing for many years. Crop management is still at the center of all the capital investment and research attention going into AI and machine learning in agriculture.
New types of remote sensors, satellites, drones, tractors, water management tools, and soil monitoring systems are currently being developed to allow growers to benefit from tech
An important thing to note is that machine learning is only as good as it is taught, and programmers work hard to teach these machines. Programmers can be informed by growers and packers to make the perfect AI tool. With this information and a finished product AI can help customers time and save money.
Applications of Machine Learning in Agriculture
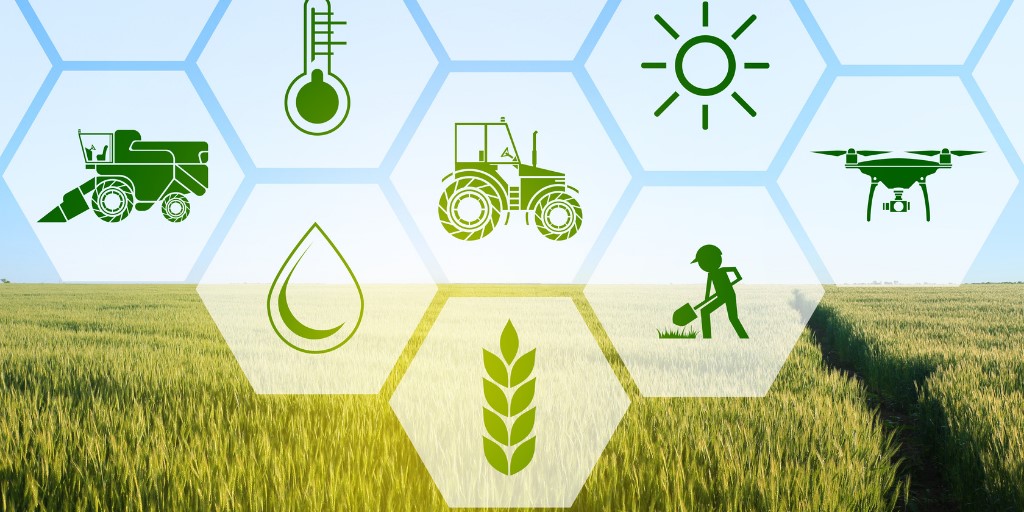
As far as crop management goes, there are five main areas using machine learning:
- Yield prediction
- Disease detection
- Weed detection
- Crop recognition
- Crop quality
Yield Prediction
In a recent scholarly review, researchers found that "yield prediction is one of the most important and challenging topics in modern agriculture." Accurate yield prediction is a complex process that has to consider many different factors . Environmental factors, management practices, genotypes, and phenotypes all interact to affect yield. Machine learning algorithms can help keep track of all that and give farmers an accurate picture of the quality of the harvest before it's in the processing facility.
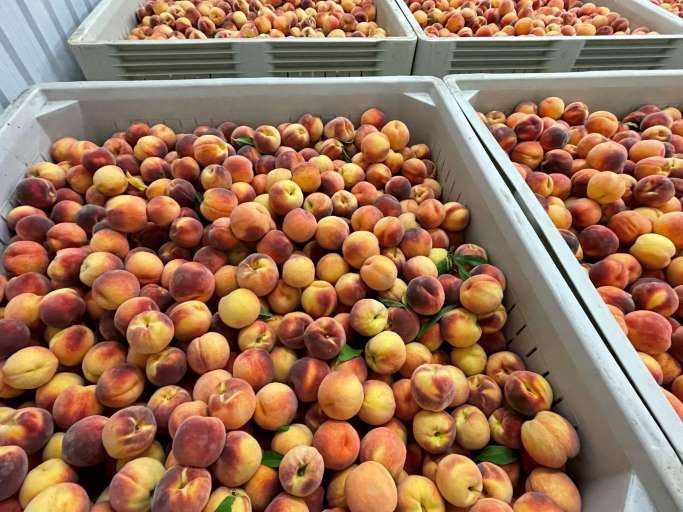
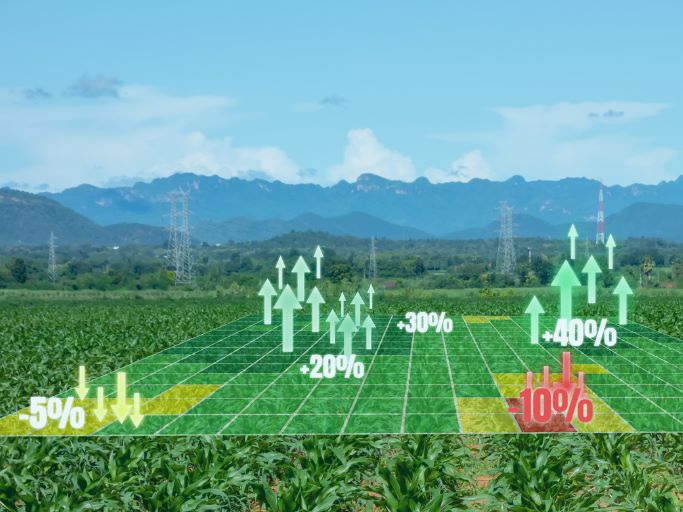
Disease and Pest Detection
Crop diseases deteriorate yield, too. So, sensing, diagnosing, and alerting the farmer to problems is also a huge help. Scientists and growers traditionally conduct disease detection with boots on the ground. Remote sensing technologies combined with numerous images of diseased and healthy plants are paving the way for new machine learning tools.
This is also known as “computer vision” and is a branch of computer image processing.
We see more machine learning algorithms working on insect and pest identification, especially in remote traps.
Weed Detection
Similarly, machine learning algorithms coupled with imaging processing technologies allow for real-time identification and mapping of target weeds. This helps with applying herbicides to specific zones instead of over-spraying fields.
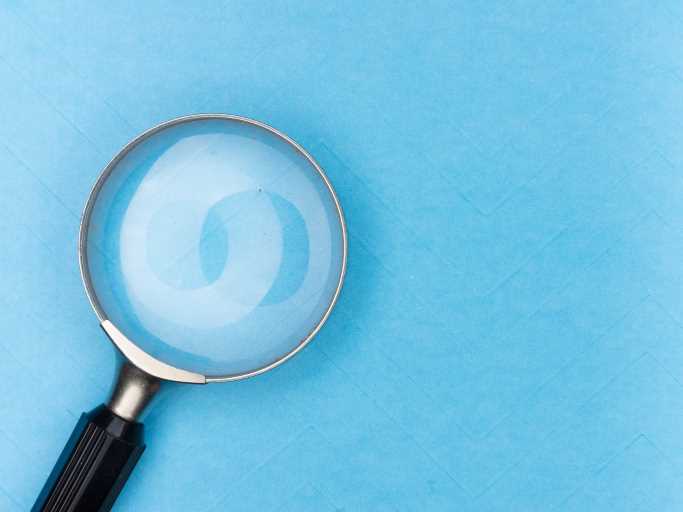
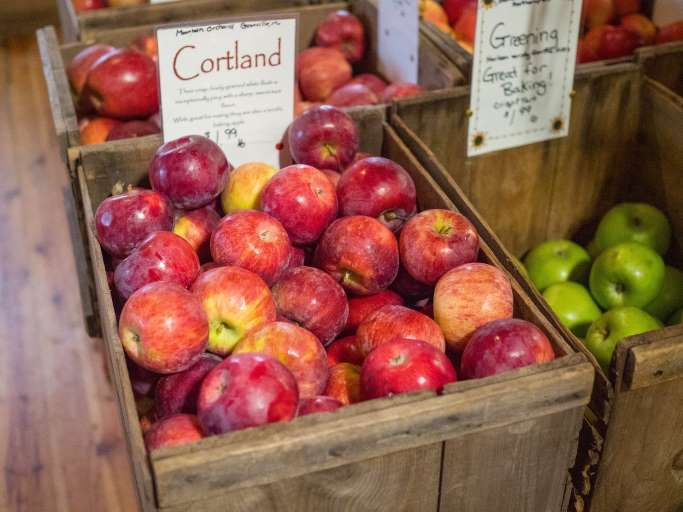
Crop Recognition
Crop recognition is a very popular application of AI in agriculture. Foliage centered plant recognition using computer vision seems to be the most common approach. Massive image datasets are now publicly available for leaf characteristics like color, shape, and texture. They use satellite images, planes, and drones to identify crops and plants.
Crop quality
Many factors go into crop quality. For example, a grower must assess the firmness of a fruit’s flesh, soluble solids content, and skin color at harvest time and beware of this all through the cold chain to the consumer. The timing of harvesting also affects the quality of the product. Machine learning algorithms help handle all this data and develop "decision support systems" to help the production teams maximize profits
Another branch of computer vision in agriculture is used in the processing plant. This type of machine learning is used in the processing plant. It can identify defects and sort the produce by weight, color, size, and ripeness. Combined with proper mechanical equipment, they can provide substantial time savings and significantly reduce the time it takes for crops to arrive at the supermarket.
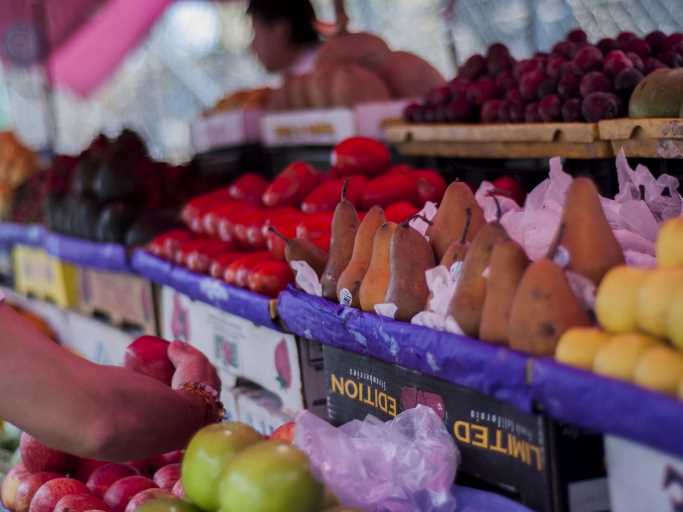
Examples of Agtech Companies in the Machine Learning Space
The development of AI and computer vision technologies is making these tools more and more accessible to smaller farms. Here's a quick rundown of current technologies available right now.
Companies Focusing on Yield Prediction
- Aibono
- Aibono's AI-powered precision farming solutions help farmers use analytics, the Internet, and machine learning to improve yield. The company synchronizes complex math and predictive harvesting using supermarket consumption data. The method reduces the likelihood of rotting produce and enables farmers to get higher profits with less waste.
- VineView
- Focusing on improving crop yields and preventing crop yields.
- VineView's drone imaging technology is designed to monitor the health of high value crops. The company uses a drone system, specialized camera equipment, and imaging processing to provide crop information.
Companies Focusing on Disease Detection.
- PlantVillage
- Triple A model (Algorithmic Agricultural Advice) that works to increase the yield and make crops profitable for millions of African farmers. They use machine learning models that recognize diseases on crops. This system was tested against expert agronomists even and won.
Companies Focusing on Weed Detection and Elimination
- FarmWise
- The FarmWise robot mechanically removes individual weeds with subinch precision. Fleets of automated weeders work together to learn what is a weed and what is a crop. They have already been used on large vegetable farms across California and Arizona for three commercial seasons.
- Blue River Technology
- Blue River, an Agtech developer since 2017, created the technology behind John Deere's See & Spray Ultimate smart sprayer to help minimize the overuse of chemicals on crops.
Companies Focusing on Crop Recognition
- Descartes Labs
- Is associated with AI labs at Los Alamos, this AI lab focuses on more than just crop recognition to include forest and natural landscapes. This company has had some success tacking and shutting down illegal deforestation that disrupts legal farms.
Companies Focusing on Crop Quality
- Croptracker
- Croptracker leads the field in computer vision for harvest quality, loss prevention, and maximum profit per harvest. This tech uses off the shelf LiDAR (laser) technology inside iPads and iPhones. With HQV 3.0 software, growers can quickly scan fruits of all sizes and shapes and receive highly accurate results in seconds.
- Hortau
- Hortau's irrigation management system has been around for 20 years and innovates with machine learning. They use sensors and AI technology that can track the amount of water available to plants. This tech is extremely useful in dry growing areas where water conservation is needed.
- Iron Ox
- Iron Ox uses robotics and AI to provide plants grown in controlled environments with the exact amount of sun, water, and nutrients needed. The company's approach to precision farming uses less water, energy, and CO2 while improving food quality.
- Trace Genomics
- Trace Genomics uses broken down DNA from the soil to create a biological and chemical profile of growing areas.
Key Takeaways:
- Machine learning is changing every industry, and agricultural leaders need to understand the basic principles, the potential, and the limitations.
- There are five main areas using machine learning:
- Yield prediction
- Disease detection
- Weed detection
- Crop recognition
- Crop quality
- AI in agricultural operations is not limited to these five operations. Though most research and development currently focuses on these five areas.
- With AIs improving in accuracy every day and more and more farmers are getting comfortable with advanced technology in their operations. A significant increase in the adoption of these technologies is inevitable
Croptracker develops agtech solutions for industry leaders. Located in Eastern Ontario, Canada, our mission is to make crop production safer, more efficient, and more profitable. Designed in partnership with fresh market producers and distributors, every tool we create is based on direct industry feedback. Croptracker’s award-winning farm software optimizes recordkeeping, labor tracking, production and cost management. Our integrative Harvest Quality Vision technology offers instant empirical analysis of crop samples exactly when you need it. Optimize your harvest season with Croptracker’s customizable solutions. Visit Croptracker.com to learn more about our products and contact us for a about app based solutions.